Bridging the In Silico-In Vitro Divide: An Integrated Platform for High-Throughput Functional Antibody Discovery
Authors: Genotic Team
The quest to harness artificial intelligence for accelerated biologics discovery, particularly in the realm of antibody engineering, holds immense promise. At Genotic, we share this vision, recognizing the potential of AI to navigate vast molecular landscapes far exceeding human capacity. However, translating computational designs into functionally validated molecules remains a formidable challenge – the persistent "translation gap." This gap stems fundamentally from the predictive limitations of current computational models, often exacerbated by the pervasive noise, incompleteness, and inherent biases within publicly available datasets. Even sophisticated models struggle to capture the complex interplay of factors governing real-world protein expression, folding, stability, and specific binding within a biological context.
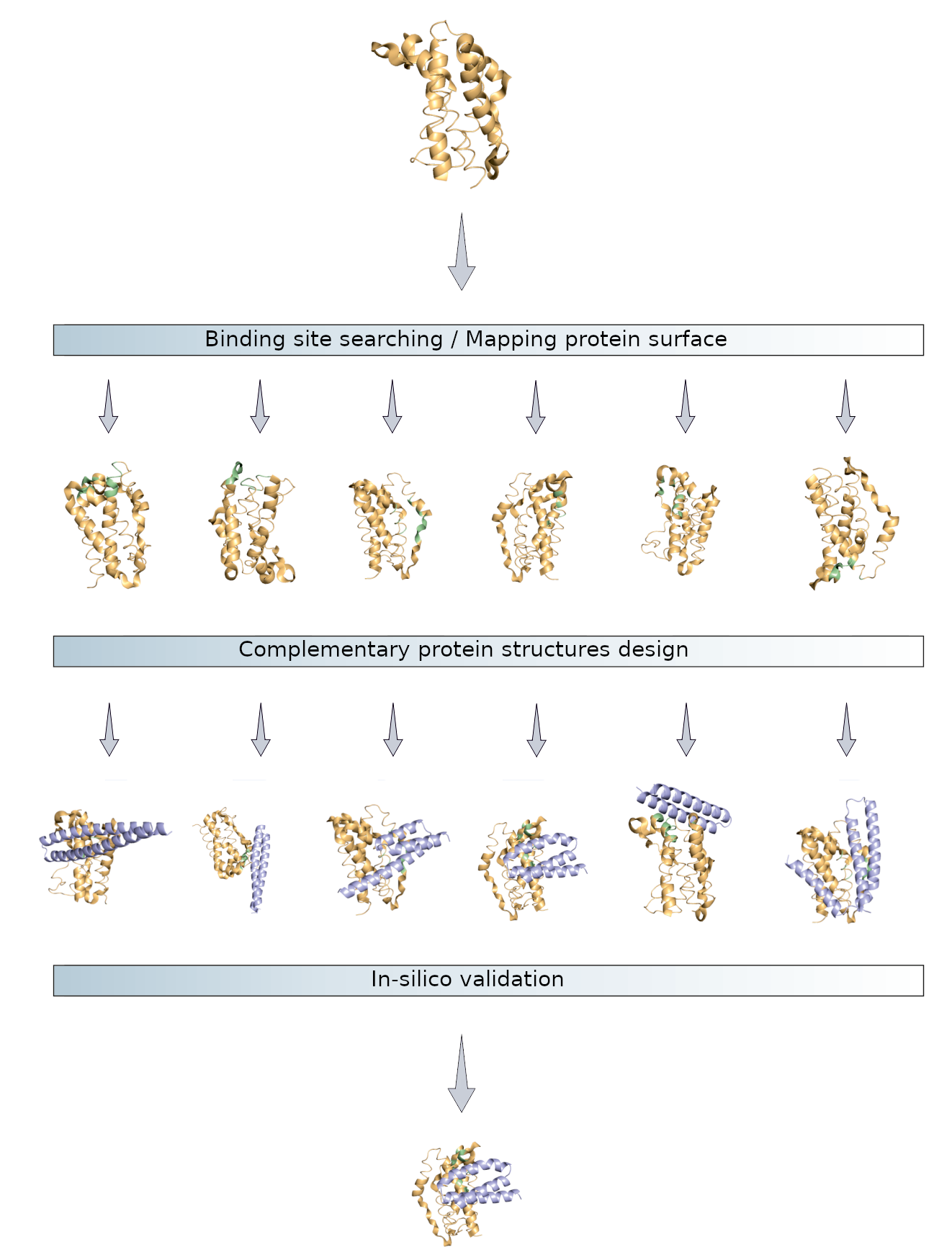
Our experience, mirroring that of many in the field, underscores that in silico success metrics frequently fail to correlate with in vitro or in vivo viability. This realization has driven our three-year effort to build beyond mere prediction and establish a truly integrated, end-to-end platform that systematically bridges computation and experimentation. We believe this holistic approach is essential for reliably delivering functional antibody candidates at scale.
The Genotic Integrated Ecosystem: Computation, Production, Validation
Our platform is architected as a unified ecosystem built upon three interconnected pillars, designed not as a linear pipeline but as a dynamic, learning system:
- Advanced Computational Design: This pillar leverages a multi-step, computationally intensive workflow powered by substantial deep learning models running on our dedicated High-Performance Computing (HPC) infrastructure. The process involves:
- Rigorous Target & Epitope Analysis: Moving beyond simple structure retrieval, we invest significant effort in curating high-quality structural representations and employ proprietary methods to identify and prioritize potential epitopes. This prioritization considers not only predicted binding affinity hotspots (integrating factors like SASA, pocket geometry, hydrophobicity, electrostatics – see Figure 2 in paper) but also epitope uniqueness across the relevant human proteome to proactively mitigate off-target risks.
- Sophisticated Binding Domain Generation: Utilizing deep learning models, we generate diverse ensembles of complementary protein scaffolds (e.g., CDRs, VHH fragments), iteratively refining them through structure prediction and sequence optimization cycles to enhance binding geometry and residue complementarity (as conceptualized in Figure 1 in paper).
- Multi-Parameter In Silico Validation Cascade: Designed sequences undergo stringent computational filtering before any wet-lab commitment. This involves predicting 3D structures, assessing structural integrity (e.g., RMSD), refining binding affinity predictions (KD, energetics), evaluating developability (predicting expression and folding success using proprietary models), performing extensive cross-reactivity checks against human protein databases, and assessing novelty and potential safety flags. Candidates must pass this multi-faceted assessment, often summarized by a composite fitness score, to proceed.
- Robust In-House Protein Production & Purification: Candidates successfully passing in silico validation seamlessly transition to our wet lab. We currently prioritize high-throughput validation using optimized E. coli expression systems for speed and cost-effectiveness, allowing rapid screening of numerous designs. Each candidate undergoes a tailored, multi-step purification process (typically IMAC followed by SEC polishing, potentially with IEX) optimized in-house to maximize yield and purity (>95% homogeneity assessed by SDS-PAGE), acknowledging the variability in expression levels observed between different designs (see Figures 3 & 4 in paper for representative chromatography).
- Rigorous Functional Validation: Purified antibodies are subjected to comprehensive functional testing. We confirm target engagement and specificity in relevant biological contexts using standard, demanding immunoassays: Immunofluorescence (IF), Flow Cytometry (FC), and, through collaboration, Immunohistochemistry (IHC) on tissue sections. Initial binding kinetics and affinity (KD) are characterized using Bio-Layer Interferometry (BLI), providing quantitative binding data.
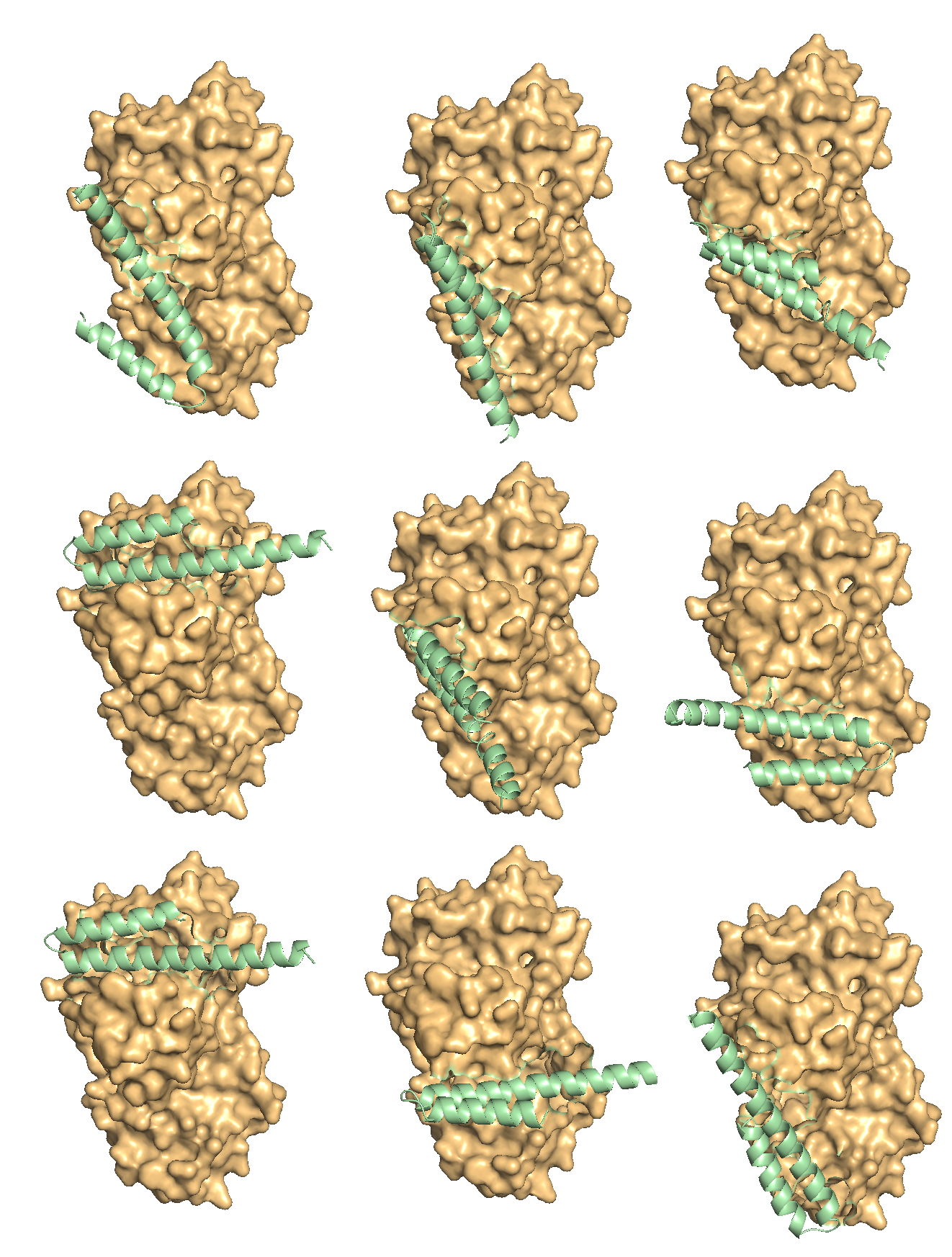
The Engine Room: Enabling Scale and Iteration with Nvidia HPC
The practical realization and scaling of this integrated vision depend critically on our substantial HPC infrastructure. This includes a dedicated cluster featuring 32x NVIDIA H100 GPUs interconnected via 1.6 Tbit/s InfiniBand, complemented by over 160 additional high-performance GPUs. This computational power is not merely supportive but enabling:
- It allows us to train and deploy the multi-billion parameter deep learning models necessary to capture the nuances of antibody structure-function relationships.
- It facilitates the massive throughput required for extensive in silico screening, simulation, and validation across thousands of potential candidates for numerous targets.
- Crucially, it powers the rapid iteration cycle, allowing us to process experimental feedback, retrain models, and generate refined designs quickly.
- It permits dynamic resource allocation, maximizing utilization and throughput across multiple parallel projects.
The Critical Feedback Loop: Learning from Experimental Reality
A cornerstone of the Genotic platform is the explicit, functional feedback loop connecting wet-lab outcomes back to the computational design engine. This is where the system truly learns and adapts. Data on successful production yields, purification profiles, stability, QC results, functional assay performance (specificity, staining patterns in IF/FC/IHC), and quantitative binding kinetics (KD values from BLI) are systematically structured and fed back.
This feedback directly informs and refines our AI models, allowing them to learn empirical correlations between sequence/structural features and real-world performance. This improves predictive accuracy for key parameters like expressibility, developability, and binding affinity, and helps guide future design strategies away from motifs associated with experimental failure. Establishing this effective loop has been a non-trivial, multi-year effort, but it is fundamental to overcoming the limitations of purely predictive approaches.
Demonstrable Success: Functional Antibodies at Scale
After three years of dedicated development, integration, and refinement, the Genotic platform is demonstrably operational and delivering results. Key achievements include:
- High Production Success: An impressive 99% of AI-designed candidates selected post-in silico filtering are successfully produced and purified in our lab, validating the predictive power of our developability assessments integrated within the design cascade.
- Significant Scale: We have designed antibody candidates for approximately 3,000 distinct targets. Antibodies for over 100 targets have been fully produced and validated through IF, FC, and/or IHC, with roughly 200 more currently in the production pipeline. This involved ~3000 individual expression cultures and thousands of purification runs.
- Functional Validation: Our produced antibodies demonstrate specific and robust performance in key immunoassays (see Figures 5-7 in paper for representative IF, FC, IHC data), confirming their utility in relevant biological applications.
- Affinity Characterization: While optimizing our BLI protocols to mitigate challenges like non-specific binding, we have successfully characterized antibodies with high affinity, achieving low nanomolar KD values (down to 10⁻⁹ M) for several candidates (see Figure 8 in paper).
Conclusion: Towards Accelerated Discovery and Personalization
The Genotic platform successfully integrates large-scale computation, automated production, and rigorous validation, governed by a critical experimental feedback loop. This synergy effectively bridges the in silico to in vitro gap, enabling the high-throughput design and generation of antibody candidates that are not only reliably producible but also functionally validated for specificity and target engagement.
While we continuously iterate to further enhance antibody affinities and developability characteristics, our platform already represents a significant step towards accelerating the discovery of impactful antibody-based tools for research, diagnostics, and potentially therapeutics. Our ultimate ambition, driven by this integrated approach and substantial computational power, is to drastically shorten development timelines, potentially enabling the rapid generation of personalized antibody solutions.
We believe this integrated, learning-based approach offers a powerful paradigm for the future of biologics discovery.
For a detailed description of our methodologies and further data, please refer to the full paper: